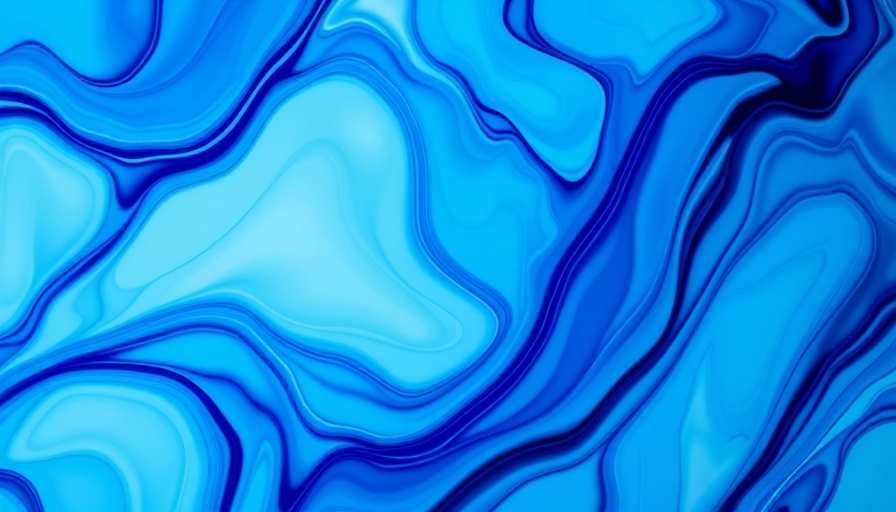
Understanding the Rise of Large Quantitative Models in AI
As technology rapidly evolves, one term gaining traction within the business community is Large Quantitative Models (LQMs). While many people are familiar with Large Language Models (LLMs), it is crucial to recognize how LQMs provide a mathematically rigorous alternative that can transform industries through data-driven insights.
The Science Behind LQMs
At their core, Large Quantitative Models utilize mathematical and statistical methods to analyze structured numerical data. According to Dave Wilson, a leading data scientist, LQMs excel in applications requiring precise predictions, such as financial forecasting, supply chain optimization, and risk assessment. Unlike their linguistic counterparts, LQMs are not just about generating text; they focus on delivering actionable insights that can directly influence strategic decision-making.
LQMs vs. LLMs: A Comparative Analysis
Understanding the distinction between LQMs and LLMs is vital for decision-makers. LLMs are trained on unstructured data like text and images, making them versatile for conversational and content-driven tasks. In contrast, LQMs are structured around numerical data, honing in on statistical analysis and predictive modeling. As Ian Quackenbos from SUSE aptly points out, "LQMs focus on numerical and quantitative analysis, processing structured data like statistics or experimental results to make predictions or simulate systems." This focus enables organizations to tap into the predictable patterns that govern financial or operational outcomes.
The Practical Applications of LQMs
With LQMs, businesses can unlock new efficiencies. For instance, an organization using LQMs for supply chain management can accurately predict disruptions and optimize inventory levels based on historical sales data and market trends. This level of precision can lead to significant cost savings and enhanced customer satisfaction.
Future Predictions: Where LQMs Are Headed
The future looks promising for LQMs as industries continue to embrace data. Most analysts agree that as computational power and mathematical methodologies advance, LQMs will become increasingly integrated into strategic planning, especially in sectors like finance, healthcare, and logistics. Dr. Stefan Leichenauer, VP of Engineering at SandboxAQ, emphasizes that leveraging quantitative principles allows businesses to innovate confidently, systematically addressing areas they may have hesitated to explore.
Challenges and Considerations
While the potential of LQMs is immense, there are challenges to be aware of. The complexity of data modeling, reliance on quality data inputs, and the requirement for specialized expertise can pose significant barriers to successful implementation. Businesses must carefully navigate these challenges to harness the full potential of LQMs.
Your Path Forward with LQMs
As the digital landscape continues to shift, integrating LQMs into your business processes could provide the analytical edge necessary for sustainable growth. It’s essential to explore how these models can be tailored to meet specific business needs, creating a roadmap for implementation. CEOs and business leaders should prioritize this integration to keep pace with competitors who are already reaping the benefits of such advanced technologies.
In summary, LQMs are not just a passing fad but rather a fundamental necessity in the toolkit of data-driven decision-making. As they complement existing data analytics capabilities, embracing them can set your business on a definitive path towards enhanced operational efficiency and success.
Write A Comment